About the Cyber-social Learning Laboratory
Mission
To design and research learning environments that establish a dialogue between artificial and human intelligence.
Precepts
Cyber-social learning connects people (who give meaning to things) with computers (which quantify and calculate these things). Such learning strives to be:
- Multilinear: traversing social networks and knowledge graphs (contrasted with linear, transmission models of standardized knowledge: teacher/text > student > one-shot test; and lock-step models knowledge progression)
- Collaborative: leveraging the social distribution of meanings, in legacy knowledge architectures/ontologies and group knowledge work (contrasted with individualized, “mentalist” pedagogies)
- Transpositional: creating a lively traffic between the sensorimotor and the cognitive, the world and its conceptual representation, human intelligence and computer intelligence; the ideal and the material (contrasted with the cognitivist biases of education, assessment, and some strands of artificial intelligence)
- Reflexive: offering small, rapid cycles of actionable human-human and human machine feedback (contrasted with long cycles, practically non-actionable cycles of traditional assessment).
- Non-scalar: nesting small scale e.g. peer-peer, one-to-one instruction, within an indefinitely scalable learning ecology (contrasted with the fixed scale of physical learning architectures and educational labor processes).
Experimental Technologies
- CGScholar: Community (social-dialogical)
- CGScholar: Creator (peer review)
- CGScholar: Analytics (educational data mining)
- CGMap (semantics meets AI)
Publications
- Cope, Bill and Mary Kalantzis, "Artificial Intelligence in the Long View: From Mechanical Intelligence to Cyber-social Systems,” Discover Artificial Intelligence, 2(13):1-18, 2022, doi: https://doi.org/10.1007/s44163-022-00029-1
- Cope, Bill and Mary Kalantzis. 2022. "The Cybernetics of Learning." Educational Philosophy and Theory:1-37. doi: https://doi.org/10.1080/001318....
- Cope, Bill and Mary Kalantzis. 2022. "The Changing Dynamics of Online Education: Five Theses on the Future of Learning." Pp. 9-33 in Foreign Language Learning in the Digital Age: Theory and Pedagogy for Developing Literacies, edited by C. Lütge. London: Routledge.
- Cope, Bill, Mary Kalantzis and Duane Searsmith. 2021. "Artificial Intelligence for Education: Knowledge and Its Assessment in Ai-Enabled Learning Ecologies." Educational Philosophy and Theory 53(12):1229-45. doi: http://doi.org/10.1080/00131857.2020.1728732
- Cope, Bill and Mary Kalantzis. 2021. "Pedagogies for Digital Learning: From Transpositional Grammar to the Literacies of Education." Pp. 34-53 in Multimodal Literacies across Digital Learning Contexts, edited by M. G. Sindoni and I. Moschini. London: Routledge.
- Kalantzis, Mary and Bill Cope. 2020a. "The Digital Learner: Towards a Reflexive Pedagogy." Pp. xviii-xxxi in Handbook of Research on Digital Learning, edited by M. Montebello. Hershey PA: IGI Global.
- Kalantzis, Mary and Bill Cope. 2020b. "After the COVID-19 Crisis: Why Higher Education May (and Perhaps Should) Never Be the Same." ACCESS: Contemporary Issues in Education 40(1):51-55. doi: https://doi.org/10.46786/ac20....
- Cope, Bill and Mary Kalantzis. 2019. "Education 2.0: Artificial Intelligence and the End of the Test."Beijing International Review of Education
1:528-43. doi: https://doi.org/10.1163/25902539-00102009.
e-Learning Ecologies
e-Learning Ecologies, the book and the videos, explore transformations in the patterns of pedagogy that accompany e-learning―the use of computing devices that mediate or supplement the relationships between learners and teachers―to present and assess learnable content, to provide spaces where students do their work, and to mediate peer-to-peer interactions. We suggest that e-learning ecologies may play a key part in shifting the systems of modern education, even as technology itself is pedagogically neutral. The result is an analytical framework with which to differentiate those aspects of educational technology that reproduce old pedagogical relations from those that are genuinely innovative and generative of new kinds of learning.
Following is a video abstract prepared for an in-person plenary presentation:
The Book: Cope, Bill and Mary Kalantzis, eds. 2016. e-Learning Ecologies: Principles for New Learning and Assessment. New York NY: Routledge.
e-Learning Ecologies - The MOOC
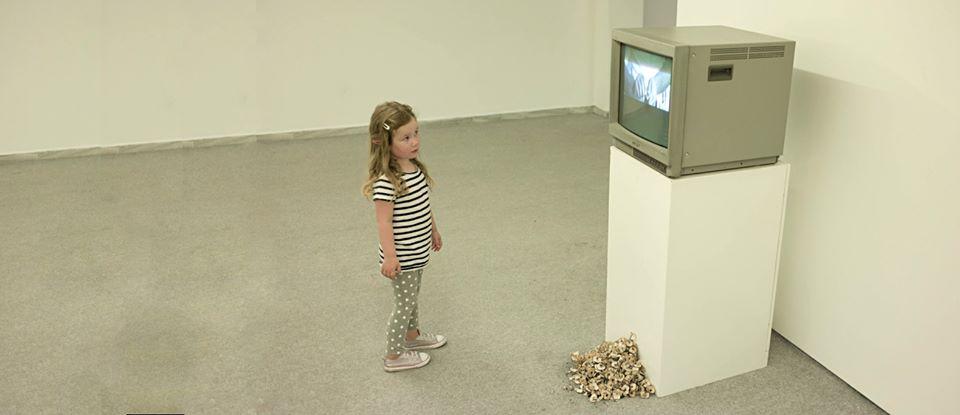
Join us in our MOOC, offered through Coursera: e-Learning Ecologies: Innovative Approaches to Teaching and Learning for the Digital Age.
For three decades and more, we’ve heard educators and technologists making a case for the transformative power of technology in learning. However, despite the rhetoric, in many sites and many ways education is still relatively untouched by technology. Even when technologies are introduced, the changes sometimes seem insignificant and the results disappointing. If the print textbook is replaced by an e-book, do the social relations of knowledge and learning necessarily change, at all or even for the better? If the pen-and-paper test is mechanized, does this change the nature of our assessment systems?
Technology, in other words, need not necessarily bring significant change or even represent a step forward in education. We propose ‘seven affordances’ of e-learning ecologies which open out genuine possibilities for what we call a ‘New Learning’ – transformative, twenty-first century learning. These affordances, if recognized and harnessed, will prepare learners for success in a world that is increasingly dominated by digital information flows, and tools for communication in the workplace, public spaces and personal life.
e-Learning Ecologies - Online Courses (The Medium is the Message!)
We also offer a course as part of our online offerings through the University of Illinois, HRD 572: e-Learning Ecologies. You can enrol in this course as a non-degree student, or take it as part of our fully online certificate, masters and doctoral programs in Learning Design and Leadership.
Teach e-Learning Ecologies in CGScholar
e-Learning Ecologies is also available in learning module format, available for anybody to teach in the CGScholar platform. Think of this as the twenty-first century substitute for the textbook...
What's the Use of Technology in Learning? Introducing Seven e-Affordances
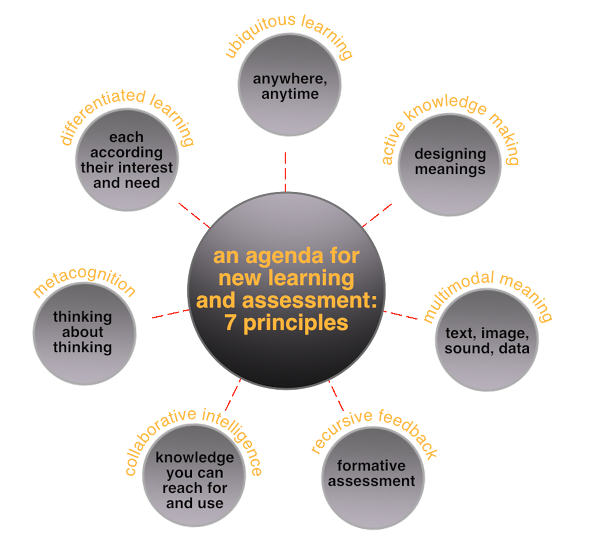
Can Education Lead Technology? The PLATO Story