Assessing Complex Epistemic Performance in Online Learning Environments
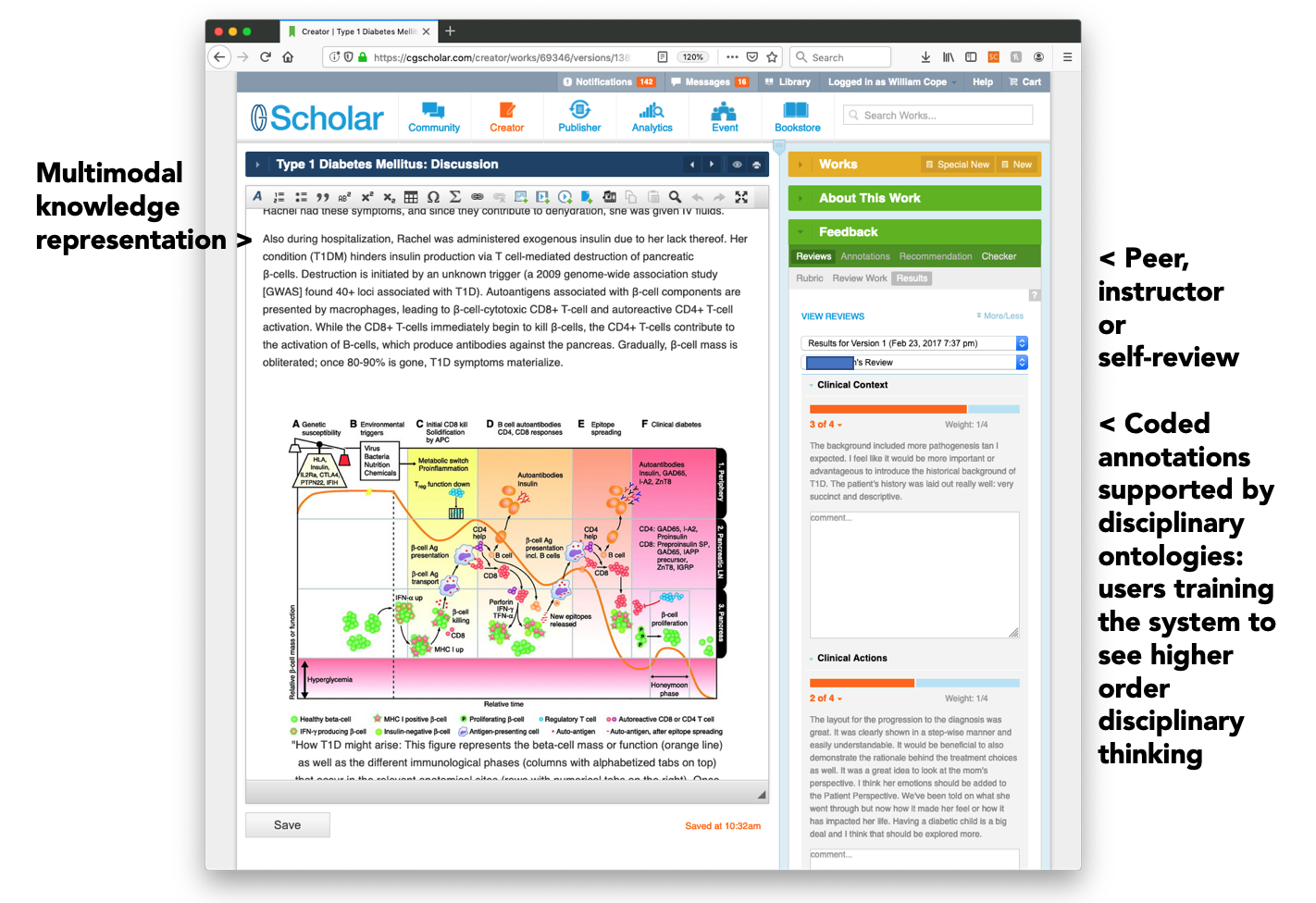
This project developed and applied innovative text mining and artificial intelligence technologies, designed to detect and offer feedback in university-level student assignments. The principal educational problem the project addressed was the inadequacy of the most common kinds of assessment:
- item-based assessments, which tend to focus narrowly on facts and correct answers to procedures; and
- summative assessments that at best offer minimal actionable feedback.
Our challenge was to develop machine-supported procedures able to assess higher level thinking, such as critical, creative or design thinking. Our solution involved students creating complex, multimodal knowledge representations including text, image, diagram, data, video etc. These ranged from documenting clinical cases in medicine and veterinary medicine, to solutions for engineering problems, to educational intervention design and evaluation. In these multimodal writing projects, students were required to be explicit about their reasoning processes and professional judgments.
Key aspects of the technologies developed in this project include:
- co-ordination of complex, multifaceted machine-learner peer and instructor feedback relationships, with actionable feedback and “big data” incremental progress visualizations for both learners and instructors
- coded annotations where the students train machine learning algorithms to detect high level thinking (e.g. evidentiary, definitional, critical, or applied thinking)
- development of innovative semantic awareness algorithms
These technologies will have wide applicability in teaching and learning contexts from Grade 4 to higher-education and workplace-based learning.
This research has been supported by the National Science Foundation, Award 1629161 to the University of Illinois at Urbana-Champaign.